Evaluating Machine Learning Models goes far beyond simply examining their accuracy. In the ever-evolving landscape of data-driven decision-making, the success of a Machine Learning Model is not solely defined by its ability to correctly classify or predict outcomes. Instead, a comprehensive understanding of the model’s performance across a multitude of metrics is essential for ensuring its real-world effectiveness and longevity.
In this article, we will delve into the intricate world of Machine Learning Model evaluation, exploring the key metrics and techniques that go beyond the simplistic measure of accuracy. Precision, recall, and the F1-score will be examined as crucial indicators of a model’s ability to balance false positives and false negatives, providing a more nuanced understanding of its strengths and weaknesses. Furthermore, the exploration of ROC curves and AUC will shed light on a model’s ability to distinguish between classes, particularly in the context of imbalanced datasets, where traditional accuracy measures may fall short.
Navigating the complexities of Machine Learning Model evaluation also necessitates a deep understanding of confusion matrices, which offer a visual representation of a model’s performance across different classes. This invaluable tool can help identify areas for improvement and guide the implementation of oversampling, undersampling, or SMOTE techniques to address imbalanced datasets.
Beyond these foundational metrics, the article will explore the importance of cross-validation methods, such as k-fold, stratified k-fold, and leave-one-out, in ensuring the robustness and generalizability of Machine Learning Models. These techniques play a crucial role in assessing a model’s performance and preventing overfitting, a common pitfall in the world of machine learning.
For regression models, the discussion will extend to MSE, RMSE, MAE, and R-squared, illuminating the nuances in evaluating the performance of models tasked with predicting continuous outcomes. Equally important is the understanding of baseline models and the art of model comparison and selection, which can greatly inform the decision-making process when it comes to deploying the most effective Machine Learning Model for a given task.
As the field of machine learning continues to evolve, the concept of concept drift and the importance of model monitoring will also be explored. These critical considerations ensure that Machine Learning Models remain relevant and responsive to changing data patterns, maintaining their efficacy over time.
By delving into these comprehensive Machine Learning Model evaluation techniques, this article aims to equip readers with the knowledge and tools necessary to move beyond the simplistic metric of accuracy and embrace a more holistic approach to assessing the true performance and impact of their Machine Learning Models.
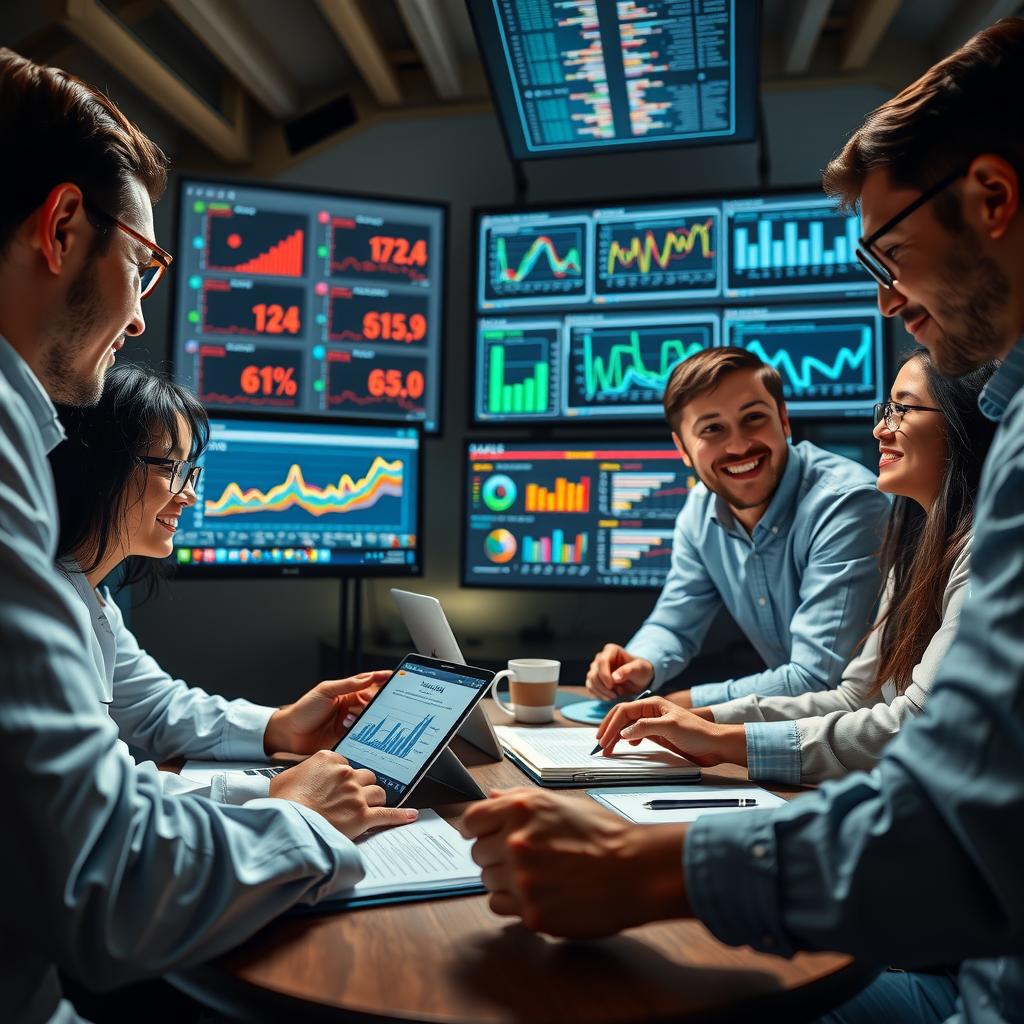
Key points:
-
Limitations of Accuracy as a Sole Evaluation Metric: Accuracy is a widely used metric for evaluating Machine Learning Models, but it may not provide a complete picture of a model’s performance, especially for imbalanced datasets or complex problem domains. Exploring alternative evaluation metrics is crucial for a more comprehensive understanding of a model’s strengths and weaknesses.
-
Understanding Precision, Recall, and the F1-score: These metrics provide a more nuanced view of a Machine Learning Model’s performance, capturing the trade-off between correctly identifying positive instances (precision) and correctly identifying all positive instances (recall). The F1-score, which combines precision and recall, offers a balanced measure of a model’s performance.
-
Receiver Operating Characteristic (ROC) Curves and Area Under the Curve (AUC): ROC curves and the AUC metric are valuable tools for evaluating the overall performance of Machine Learning Models, especially in binary classification tasks. They provide insights into the trade-off between a model’s true positive rate and false positive rate, helping to assess its discrimination ability.
-
Confusion Matrices and Their Interpretation: Analyzing the confusion matrix, which showcases a Machine Learning Model’s true positives, true negatives, false positives, and false negatives, can reveal valuable insights about a model’s performance and guide improvements.
-
Dealing with Imbalanced Datasets: Oversampling, Undersampling, and SMOTE: When working with datasets where one class is significantly more prevalent than others, traditional accuracy metrics may be misleading. Techniques like oversampling, undersampling, and SMOTE can help address this issue and ensure a more robust evaluation of Machine Learning Models.
-
Cross-Validation Techniques: K-fold, Stratified K-fold, and Leave-One-Out: Proper model evaluation requires reliable techniques to assess a Machine Learning Model’s generalization performance. Cross-validation methods, such as k-fold, stratified k-fold, and leave-one-out, can help provide an unbiased estimate of a model’s performance.
-
Evaluating Regression Models: MSE, RMSE, MAE, and R-squared: For regression tasks, evaluating Machine Learning Models requires different metrics, such as Mean Squared Error (MSE), Root Mean Squared Error (RMSE), Mean Absolute Error (MAE), and R-squared, to capture the model’s ability to accurately predict continuous target variables.
-
The Importance of Baseline Models in Evaluation: Comparing a Machine Learning Model’s performance to appropriate baseline models is crucial for determining its true value and identifying areas for improvement.
-
Techniques for Model Comparison and Selection: Employing techniques like statistical significance tests and model comparison frameworks can help data scientists make informed decisions about which Machine Learning Model to deploy, based on their unique requirements and constraints.
-
Evaluating Models in Production: Concept Drift and Model Monitoring: Ensuring the continued performance of Machine Learning Models in production requires monitoring for concept drift, where the underlying data distribution changes over time, and implementing appropriate model monitoring strategies.
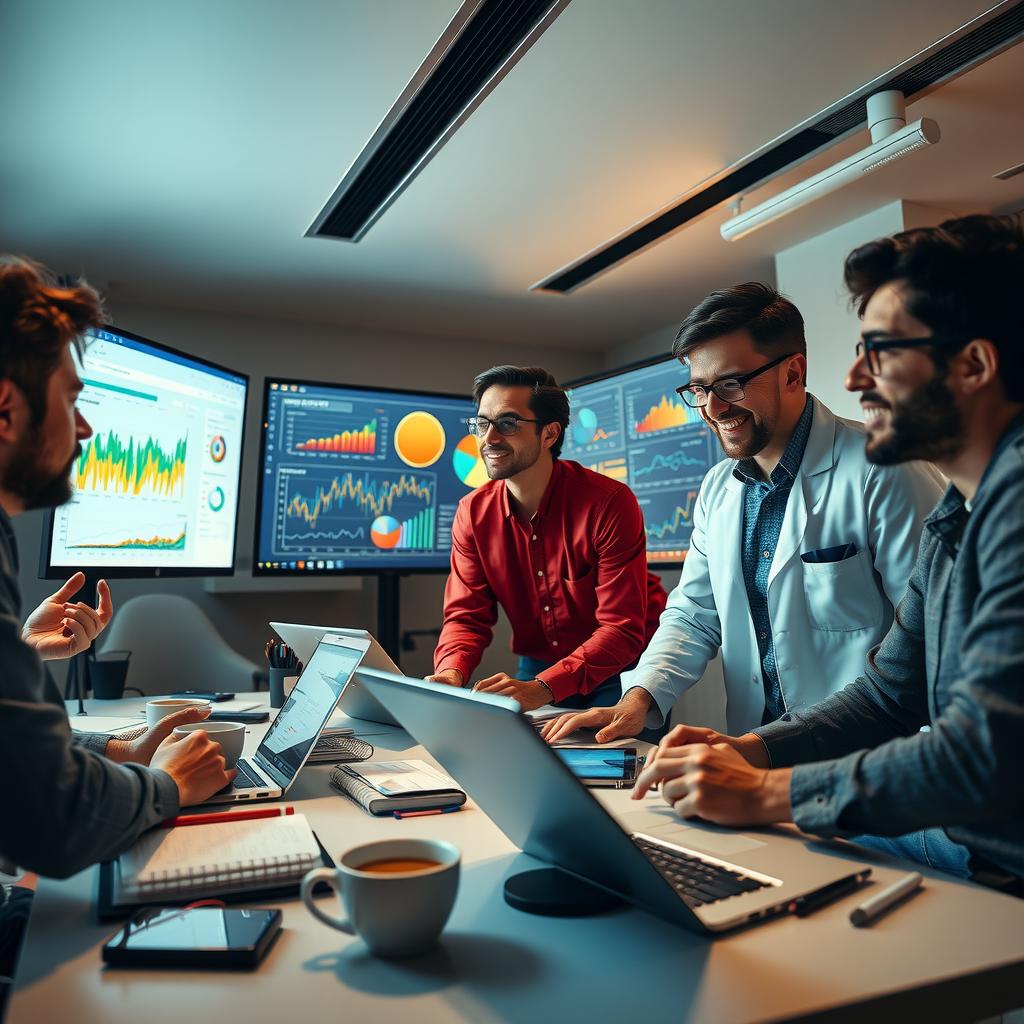
Unveiling the True Potential: Exploring Alternative Metrics for Evaluating ML Models
Beyond Accuracy: Comprehensive Model Evaluation
When it comes to evaluating the performance of Machine Learning Models, accuracy is often the go-to metric. However, in many real-world scenarios, accuracy alone may not provide a complete picture of a model’s effectiveness. In this article, we will explore a range of alternative metrics that can help unveil the true potential of your Machine Learning Models and guide you towards more informed decision-making.
Accuracy is undoubtedly an important metric, as it measures the overall correctness of a model’s predictions. However, in situations where the dataset is imbalanced, or the cost of different types of errors varies, accuracy may not be the most meaningful evaluation criterion. In such cases, metrics like precision, recall, and F1-score can provide a more comprehensive understanding of a model’s performance. Precision measures the proportion of true positives among all positive predictions, while recall measures the proportion of true positives among all actual positive instances. The F1-score combines these two metrics into a single, harmonious score, offering a more balanced evaluation.
Another valuable tool for evaluating Machine Learning Models is the Receiver Operating Characteristic (ROC) curve and the corresponding Area Under the Curve (AUC) metric. The ROC curve plots the true positive rate against the false positive rate, providing insights into the trade-off between sensitivity and specificity. The AUC, on the other hand, quantifies the overall discriminative ability of a model, making it particularly useful for binary classification tasks.
When dealing with imbalanced datasets, it’s crucial to consider alternative approaches to address the inherent class imbalance. Techniques such as oversampling, undersampling, and Synthetic Minority Over-sampling Technique (SMOTE) can help balance the dataset, leading to more reliable model evaluations and improved performance.
Beyond classification tasks, Machine Learning Models are also employed for regression problems, where metrics like Mean Squared Error (MSE), Root Mean Squared Error (RMSE), and Mean Absolute Error (MAE) become relevant. These metrics measure the average magnitude of the errors in the same units as the target variable, providing valuable insights into a model’s predictive accuracy.
When comparing the performance of multiple Machine Learning Models or evaluating the suitability of a model for a specific task, it’s important to consider techniques like cross-validation, k-fold, stratified k-fold, and leave-one-out. These methods help ensure the reliability and generalizability of the model’s performance, mitigating the risk of overfitting or biased evaluations.
It’s also crucial to understand the concept of concept drift, which describes the phenomenon where the underlying data distribution changes over time, rendering the trained Machine Learning Model less accurate. Monitoring the model’s performance and implementing strategies to detect and adapt to concept drift can help maintain the model’s effectiveness in dynamic environments.
By expanding our focus beyond the traditional accuracy metric and exploring a range of alternative evaluation techniques, we can gain a deeper understanding of the strengths, weaknesses, and suitability of our Machine Learning Models for various real-world applications. This comprehensive approach to model evaluation empowers us to make more informed decisions, optimize model performance, and unlock the true potential of our Machine Learning endeavors.
The Importance of Baseline Models and Model Comparison
When evaluating the performance of Machine Learning Models, it’s essential to consider the use of baseline models as a point of reference. Baseline models are simplistic yet reliable models that serve as a benchmark for comparison, helping to determine whether the more complex Machine Learning Models offer tangible improvements in performance.
Comparing the performance of your Machine Learning Model against appropriate baseline models can provide valuable insights. If the Machine Learning Model does not outperform the baseline model, it may indicate that the complexity of the Machine Learning Model is not justified, or that the dataset or problem at hand may not be suitable for a more sophisticated approach.
On the other hand, if the Machine Learning Model demonstrates a significant improvement over the baseline model, it reinforces the value of the Machine Learning Model and its ability to capture relevant patterns and relationships in the data. This comparison can also inform decisions about model selection, guiding you towards the most appropriate Machine Learning Model for your specific use case.
When comparing the performance of multiple Machine Learning Models, it’s important
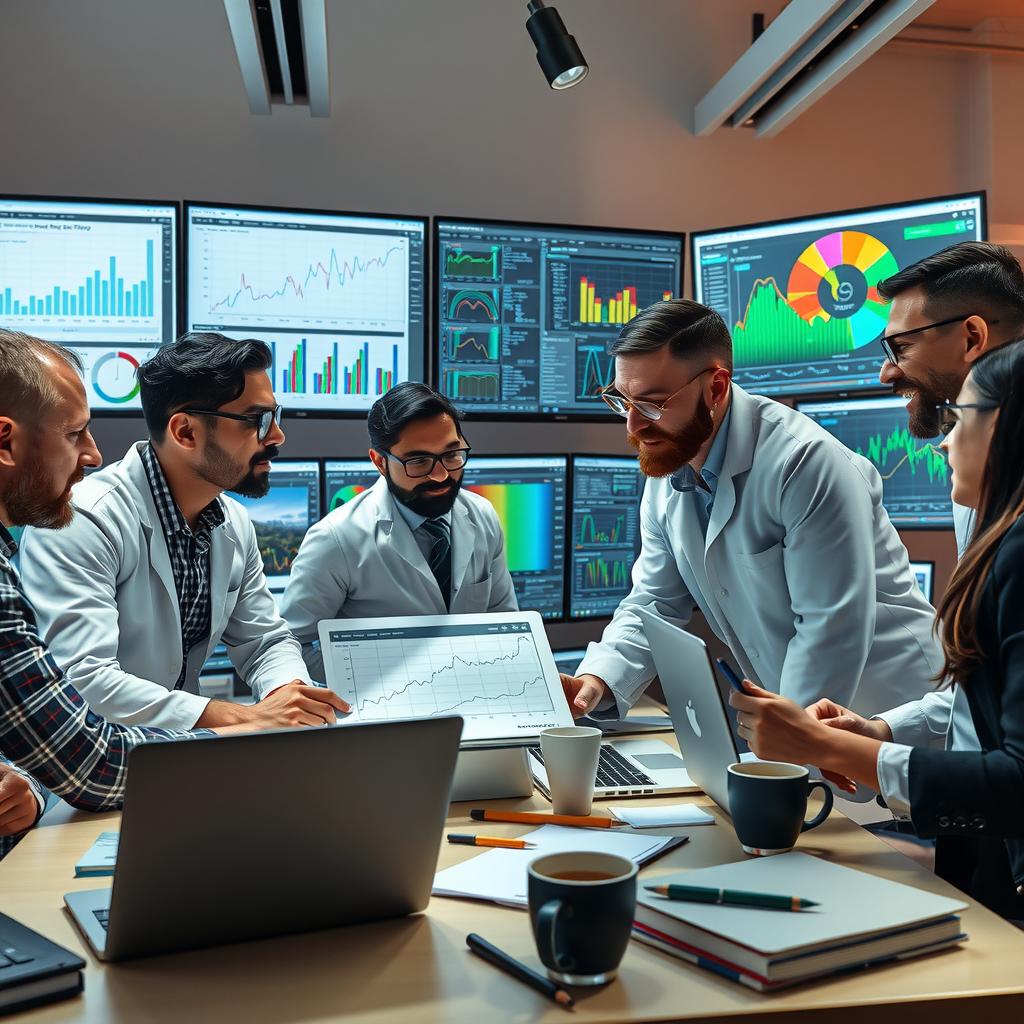
Beyond Accuracy: Unlocking the Hidden Gems
Dive into Precision
In the realm of Machine Learning Model evaluation, accuracy is often the primary metric that receives the lion’s share of attention. While accuracy is undoubtedly important, it is merely one facet of a multifaceted evaluation process. To truly unlock the hidden potential of your Machine Learning Model, it is crucial to dive deeper and explore a wider range of evaluation metrics.
One of the key areas to consider beyond accuracy is precision. Precision measures the proportion of true positive predictions out of all the positive predictions made by the model. In other words, it quantifies the model’s ability to correctly identify positive instances. This metric is particularly important when dealing with imbalanced datasets, where the number of positive and negative instances differs significantly. In such scenarios, a model might achieve high accuracy by simply predicting the majority class, but precision would reveal if the model is genuinely effective in identifying the minority class.
Another important metric to consider is recall, which measures the proportion of true positive predictions out of all the actual positive instances. Recall reflects the model’s ability to correctly identify all the positive instances, even if it also predicts some false positives. A balance between precision and recall is often sought, and the F1-score, which combines these two metrics, provides a comprehensive evaluation of the model’s performance.
Visualization tools, such as ROC curves and AUC, can also provide valuable insights into the model’s performance. ROC curves plot the true positive rate against the false positive rate, while AUC measures the area under the ROC curve, indicating the model’s ability to distinguish between positive and negative instances.
Furthermore, confusion matrices offer a detailed breakdown of the model’s performance, showing the true positives, true negatives, false positives, and false negatives. This information can be particularly useful when dealing with imbalanced datasets, as it allows you to identify where the model is struggling and make informed decisions about potential remedies, such as oversampling or undersampling techniques like SMOTE.
In the realm of regression models, additional metrics like MSE (Mean Squared Error), RMSE (Root Mean Squared Error), MAE (Mean Absolute Error), and R-squared provide valuable insights into the model’s ability to accurately predict continuous target variables.
Ultimately, a comprehensive Machine Learning Model evaluation goes beyond simply measuring accuracy. By exploring a diverse set of evaluation metrics, you can uncover the hidden gems within your model, identify its strengths and weaknesses, and make informed decisions about model selection, tuning, and deployment. This holistic approach ensures that your Machine Learning Model not only achieves high accuracy but also delivers meaningful and reliable predictions.
Navigating the Complexities of Model Evaluation
As the field of Machine Learning continues to evolve, the landscape of model evaluation has become increasingly complex. Beyond the traditional accuracy metric, Machine Learning practitioners must navigate a myriad of evaluation metrics, each offering unique insights into the performance of their Machine Learning Models.
One of the key challenges in model evaluation is dealing with imbalanced datasets, where the distribution of positive and negative instances is skewed. In such scenarios, accuracy alone can be misleading, as a model might achieve high accuracy simply by predicting the majority class. Precision and recall become crucial metrics, as they offer a more nuanced understanding of the model’s ability to correctly identify positive instances, even in the face of class imbalance.
The F1-score, which combines precision and recall, provides a well-rounded evaluation of the model’s performance, balancing the importance of correctly identifying positive instances while also considering the model’s ability to avoid false positives.
Visualization tools, such as ROC curves and AUC, offer a powerful way to assess the model’s performance across a range of classification thresholds. These tools can help Machine Learning practitioners identify the optimal trade-off between true positive and false positive rates, informing their decisions about model selection and deployment.
In the realm of regression models, additional metrics like MSE, RMSE, MAE, and R-squared provide valuable insights into the model’s ability to accurately predict continuous target variables. Understanding the strengths and limitations of these metrics can inform model selection, hyperparameter tuning, and the identification of appropriate baseline models for comparison.
As Machine Learning models are deployed in real-world scenarios, the challenge of *concept drift
Recall: Uncovering the Essence of Model Performance
Precision, Recall, and the Elusive Balance
When evaluating the performance of a Machine Learning Model, accuracy is often the first metric that comes to mind. However, in many real-world scenarios, the true test of a model’s effectiveness lies beyond this single measure. Recall, a lesser-known but equally crucial metric, sheds light on the model’s ability to identify all relevant instances, even in the face of imbalanced datasets.
Imagine a scenario where a Machine Learning Model is tasked with detecting fraudulent transactions. In this context, accurately identifying all fraudulent transactions (high recall) is far more critical than simply achieving a high overall accuracy. After all, missing a single fraudulent transaction can have severe consequences. By delving into recall, we gain a deeper understanding of the model’s performance in this crucial aspect, ensuring that it not only performs well but also fulfills its intended purpose.
Balancing Precision and Recall: The F1-Score Revelation
While recall is undoubtedly important, it is often at odds with precision, the model’s ability to avoid false positives. The true power of model evaluation lies in finding the right balance between these two metrics, a challenge that the F1-score aims to address.
The F1-score is a harmonic mean of precision and recall, providing a single metric that captures the model’s overall performance. By considering both the ability to identify all relevant instances (recall) and the accuracy of those identifications (precision), the F1-score offers a more comprehensive assessment of the Machine Learning Model’s effectiveness.
Visualizing Performance: ROC Curves and AUC
Looking beyond individual metrics, Machine Learning Models can be further evaluated through the lens of ROC (Receiver Operating Characteristic) curves and AUC (Area Under the Curve). These powerful tools enable a more nuanced understanding of a model’s performance across a range of threshold values, allowing for informed decisions on the optimal balance between precision and recall.
ROC curves plot the true positive rate (related to recall) against the false positive rate, while the AUC measure the overall discriminative capability of the model. By analyzing these visualizations, practitioners can gain valuable insights into the model’s ability to distinguish between positive and negative instances, informing their decision-making process and guiding further model refinement.
Navigating Imbalanced Datasets: Overcoming Challenges
In many real-world applications, datasets are often imbalanced, with one class significantly outnumbering the other. This can pose a challenge for traditional Machine Learning Models, as they may become biased towards the majority class, compromising recall for the minority class.
To address this issue, techniques such as oversampling, undersampling, and SMOTE (Synthetic Minority Over-sampling Technique) can be employed to balance the dataset, ensuring that the Machine Learning Model is trained to recognize patterns in both the majority and minority classes effectively.
Evaluating Model Performance: Going Beyond the Basics
While accuracy, precision, and recall provide a solid foundation for evaluating Machine Learning Models, there are additional metrics that may be relevant depending on the specific use case. For regression tasks, mean squared error (MSE), root mean squared error (RMSE), and mean absolute error (MAE) offer valuable insights into the model’s ability to predict continuous target variables.
Furthermore, the concept of baseline models and model comparison is crucial in assessing the true performance of a Machine Learning Model. By benchmarking against simpler models or industry-standard approaches, practitioners can determine whether the Machine Learning Model under evaluation truly adds value and outperforms other available solutions.
In conclusion, the evaluation of Machine Learning Models goes far beyond a single accuracy metric. By delving into recall, precision, F1-score, ROC curves, and AUC, as well as addressing challenges posed by imbalanced datasets and exploring additional performance metrics, practitioners can gain a comprehensive understanding of their models’ strengths, weaknesses, and overall effectiveness. This holistic approach ensures that Machine Learning Models are not only technically sound but also aligned with the real-world objectives they are designed to achieve.
F1-score
Measuring Model Performance Beyond Accuracy
In the realm of machine learning, the evaluation of model performance goes far beyond the simple metric of accuracy. While accuracy is a valuable measure, it often fails to capture the nuances of model behavior, particularly in scenarios with imbalanced datasets or complex classification tasks. One such metric that provides a more comprehensive assessment is the F1-score.
The F1-score is the harmonic mean of precision and recall, two essential metrics in the evaluation of classification models. Precision represents the proportion of true positive predictions among all positive predictions made by the model, while recall measures the proportion of true positive predictions out of all actual positive instances in the data. By combining these two metrics, the F1-score offers a balanced evaluation that considers both the model’s ability to correctly identify positive instances and its propensity to avoid false positives.
The formula for the F1-score is:
F1-score = 2 (Precision Recall) / (Precision + Recall)
The F1-score ranges from 0 to 1, with 1 indicating a perfect balance between precision and recall. This metric is particularly useful in scenarios where the cost of false positives and false negatives are equally important, or when the dataset is imbalanced, and accuracy alone may not provide a complete picture of the model’s performance.
Interpreting the F1-score
The F1-score can be interpreted as follows:
- F1-score = 1: The model has perfect precision and recall, meaning it correctly identifies all positive instances and has no false positives.
- F1-score = 0: The model has either no true positives or all its predictions are false positives.
- F1-score close to 1: The model has a good balance between precision and recall, indicating high overall performance.
- F1-score close to 0: The model has poor precision and recall, suggesting it is not performing well.
The F1-score is particularly useful in situations where the dataset is imbalanced, and accuracy alone may not provide a complete picture of the model’s performance. In such cases, the F1-score can help identify models that strike the right balance between correctly identifying positive instances and minimizing false positives.
Applying the F1-score in Model Evaluation
The F1-score is a versatile metric that can be applied to a wide range of Machine Learning Model classification tasks, from binary classification to multi-class problems. It is often used in conjunction with other evaluation metrics, such as Receiver Operating Characteristic (ROC) curves and Area Under the Curve (AUC), to provide a more holistic view of model performance.
By considering the F1-score in addition to accuracy, data scientists and machine learning practitioners can make more informed decisions about model selection, optimization, and deployment, ensuring that the chosen Machine Learning Model not only performs well on the data but also generalizes effectively to real-world scenarios.
Machine Learning Model Evaluation: Beyond Accuracy
Comprehensive Model Evaluation Metrics
While accuracy is a commonly used metric for evaluating the performance of Machine Learning Models, it often fails to provide a complete picture, especially when dealing with complex or imbalanced datasets. Precision, recall, and the F1-score offer a more nuanced understanding of a model’s performance, accounting for both false positives and false negatives. ROC curves and AUC (Area Under the Curve) provide insights into a model’s trade-off between true positive and false positive rates, while confusion matrices can reveal specific misclassification patterns. These metrics are particularly important when dealing with imbalanced datasets, where techniques like oversampling, undersampling, and SMOTE (Synthetic Minority Over-sampling Technique) can be employed to address class imbalance.
Advanced Evaluation Techniques for Machine Learning Models
Beyond classification-based metrics, regression models can be evaluated using metrics like MSE (Mean Squared Error), RMSE (Root Mean Squared Error), MAE (Mean Absolute Error), and R-squared. These metrics help assess the model’s ability to accurately predict continuous target variables. Additionally, the use of baseline models and model comparison techniques can provide valuable insights into the relative performance of Machine Learning Models. Cross-validation methods, such as k-fold, stratified k-fold, and leave-one-out, can help ensure the robustness and generalizability of model performance.
Furthermore, it is crucial to consider the issue of concept drift, where the underlying data distribution changes over time, affecting the model’s performance. Model monitoring can help detect and address such changes, ensuring that Machine Learning Models continue to perform well in real-world scenarios.
By incorporating these advanced evaluation techniques, practitioners can gain a more comprehensive understanding of their Machine Learning Models’ strengths, weaknesses, and suitability for specific applications. This holistic approach to model evaluation can lead to better-informed decisions, more robust model selection, and ultimately, more effective Machine Learning solutions.
Unraveling the Complexities: Decoding Confusion Matrices and ROC Curves
Unleashing the Power of Visualization Tools
Evaluating the performance of a Machine Learning Model goes beyond simply measuring its accuracy. To truly understand the intricacies of a model’s decision-making process, data scientists and machine learning practitioners rely on powerful visualization tools, such as confusion matrices and ROC (Receiver Operating Characteristic) curves. These tools provide invaluable insights that can help refine and optimize the Machine Learning Model for better real-world performance.
A confusion matrix is a table that visualizes the performance of a Machine Learning Model on a set of test data, where the actual and predicted classes are compared. By analyzing the matrix, you can gain a deeper understanding of the model’s strengths and weaknesses, such as its ability to correctly identify true positives and true negatives, as well as its propensity for false positives and false negatives. This information is crucial in scenarios where the cost of different types of errors varies, such as in medical diagnostics or fraud detection.
Complementing the confusion matrix, the ROC curve is a plot that visualizes the trade-off between the true positive rate (sensitivity) and the false positive rate (1 – specificity) of a Machine Learning Model across different classification thresholds. The area under the ROC curve (AUC-ROC) is a widely used metric that provides a comprehensive evaluation of the model’s performance, indicating its ability to distinguish between different classes. ROC curves are particularly useful for evaluating the performance of Machine Learning Models in binary classification tasks, where the goal is to predict whether an instance belongs to one of two classes.
In situations where the dataset is imbalanced, with a significant disparity in the number of instances between different classes, these visualization tools become even more crucial. Confusion matrices and ROC curves can help identify and address issues such as class imbalance, which can lead to biased model performance. Techniques like oversampling, undersampling, and synthetic data generation (e.g., SMOTE) can be employed to mitigate the effects of imbalanced datasets and improve the Machine Learning Model’s overall performance.
Additionally, these visualization tools are invaluable in the context of model selection and comparison. By comparing the confusion matrices and ROC curves of different Machine Learning Models, you can make informed decisions about which model best suits your specific use case and requirements. This analysis can also help identify potential areas for model improvement, such as the need for feature engineering, hyperparameter tuning, or the incorporation of additional data sources.
In conclusion, Machine Learning Model evaluation goes beyond simply measuring accuracy. By leveraging the power of confusion matrices and ROC curves, data scientists and machine learning practitioners can gain deeper insights into a model’s decision-making process, identify and address performance issues, and make informed decisions about model selection and optimization. These visualization tools are essential in the pursuit of building robust, reliable, and high-performing Machine Learning Models.
Navigating the Intricacies of Model Evaluation Metrics
While accuracy is a commonly used metric for evaluating Machine Learning Models, it may not always provide a comprehensive picture of a model’s performance, especially in scenarios with imbalanced datasets or varying costs of different types of errors. To gain a more nuanced understanding, data scientists and machine learning practitioners often turn to a suite of evaluation metrics, each shedding light on different aspects of a model’s behavior.
Precision, Recall, and F1-Score are key metrics that provide a more holistic assessment of a Machine Learning Model’s performance. Precision measures the proportion of true positives among all positive predictions, while Recall quantifies the model’s ability to identify all true positives. The F1-Score, the harmonic mean of Precision and Recall, offers a balanced metric that considers both the model’s ability to make accurate predictions and its capacity to identify all relevant instances.
In situations where the cost of different types of errors varies, the ROC curve and the Area Under the Curve (AUC-ROC) become particularly valuable. The ROC curve visualizes the trade-off between the true positive rate and the false positive rate, allowing for a more nuanced evaluation of the model’s performance across different classification thresholds. The AUC-ROC metric, which ranges from 0.5 (random guessing) to 1 (perfect classification), provides a comprehensive assessment of the model’s ability to distinguish between different classes.
Beyond binary classification tasks, evaluating the performance of Machine Learning Models in regression
Conquering Imbalanced Datasets: Strategies for Robust Evaluation
Explore Techniques Like Oversampling
When dealing with imbalanced datasets, where one class is significantly underrepresented compared to the others, traditional machine learning models can struggle to learn the underlying patterns effectively. This can lead to biased predictions and poor overall performance, particularly on the minority class. One powerful technique to address this challenge is oversampling.
Oversampling involves increasing the representation of the minority class in the training data, effectively balancing the class distribution. This can be accomplished through various methods, such as Synthetic Minority Over-sampling Technique (SMOTE), which generates synthetic examples of the minority class by interpolating between existing instances. Another approach is random oversampling, where instances of the minority class are simply duplicated to achieve the desired balance.
The benefits of oversampling extend beyond just improving the Machine Learning Model‘s accuracy on the minority class. By balancing the class distribution, the model can learn more robust and generalizable patterns, reducing the risk of overfitting to the majority class. This, in turn, can lead to improved F1-score, precision, and recall metrics, providing a more comprehensive evaluation of the Machine Learning Model‘s performance.
When implementing oversampling, it’s crucial to ensure that the synthetic or duplicated examples do not introduce additional noise or biases into the training data. Techniques like Borderline-SMOTE can help generate more informative synthetic examples by focusing on the boundaries between classes. Additionally, it’s essential to carefully monitor the performance of the Machine Learning Model on both the majority and minority classes, as oversampling can sometimes lead to overfitting on the minority class.
To further enhance the robustness of the Machine Learning Model, it’s recommended to combine oversampling with other techniques, such as undersampling the majority class or using class weights to adjust the importance of each class during training. Additionally, cross-validation strategies, like stratified k-fold, can help ensure that the Machine Learning Model is evaluated on a representative sample of the imbalanced dataset.
By leveraging oversampling and other strategies to address imbalanced datasets, researchers and practitioners can develop Machine Learning Models that are more accurate, reliable, and equitable across all classes, paving the way for more robust and trustworthy predictions.
Handling Imbalance with Undersampling
In addition to oversampling the minority class, another effective technique for conquering imbalanced datasets is undersampling the majority class. This approach reduces the number of instances from the majority class, again aiming to balance the class distribution and improve the Machine Learning Model‘s performance.
One common undersampling method is random undersampling, where a subset of the majority class instances is randomly selected and removed from the training data. This simple approach can be effective, but it may result in the loss of potentially valuable information from the majority class.
To address this, more advanced undersampling techniques have been developed, such as Tomek Links and Edited Nearest Neighbor (ENN). Tomek Links identify and remove majority class instances that are close to the decision boundary, while ENN removes majority class instances that are misclassified by their nearest neighbors.
By combining oversampling and undersampling techniques, researchers and practitioners can achieve an optimal balance in the dataset, maximizing the Machine Learning Model‘s ability to learn from the available information. This approach, known as hybrid sampling, can lead to significant improvements in the Machine Learning Model‘s performance on imbalanced datasets.
It’s important to note that the choice of oversampling and undersampling techniques should be tailored to the specific problem and dataset at hand. The effectiveness of these methods can vary depending on the underlying data distribution, the degree of imbalance, and the complexity of the Machine Learning Model being used.
Evaluating Model Performance Beyond Accuracy
When dealing with imbalanced datasets, traditional accuracy-based metrics may not provide a complete picture of the Machine Learning Model‘s performance. Instead, it’s crucial to consider a range of evaluation metrics that capture different aspects of the model’s behavior.
One important metric is the F1-score, which combines precision and recall into a single value. The F1-score is particularly useful for imbalanced datasets, as it provides a balanced measure of the model’s ability to correctly identify both the majority and minority classes.
Another valuable metric
Undersampling: Balancing Imbalanced Datasets
Addressing Class Imbalance through Undersampling
Undersampling is a powerful technique employed in machine learning to address the challenge of class imbalance, where one class dominates the dataset significantly compared to the other class(es). This scenario can lead to poor model performance, as the model may become biased towards the majority class and fail to accurately predict the minority class. Undersampling aims to alleviate this issue by reducing the number of samples in the majority class, thereby creating a more balanced dataset.
The primary objective of undersampling is to reduce the size of the majority class while preserving the essential characteristics of the dataset. This is achieved by selectively removing instances from the majority class, either randomly or based on specific criteria. Random undersampling is a simple approach where a subset of the majority class is randomly selected and removed from the dataset. More sophisticated techniques, such as Tomek Links or Condensed Nearest Neighbor, identify and remove majority class instances that are close to the decision boundary or are redundant, thereby retaining the most informative samples.
Undersampling can be particularly beneficial when dealing with highly imbalanced datasets, where the minority class represents a small fraction of the overall data. By reducing the majority class, the model is forced to focus on learning the patterns in the minority class, which can lead to improved performance in terms of precision, recall, and F1-score. Additionally, undersampling can help reduce the computational complexity of the machine learning model, as it operates on a smaller dataset.
It is important to note that undersampling should be applied with caution, as removing too many instances from the majority class may result in the loss of valuable information. It is often recommended to combine undersampling with other techniques, such as oversampling or SMOTE (Synthetic Minority Over-sampling Technique), to achieve a better balance between the classes and improve the overall model performance.
Evaluating the Impact of Undersampling
To assess the effectiveness of undersampling, it is crucial to evaluate the model’s performance using a range of metrics beyond just accuracy. Precision, recall, and F1-score are commonly used metrics that provide a more comprehensive understanding of the model’s ability to correctly identify both the majority and minority classes. Additionally, ROC (Receiver Operating Characteristic) curves and AUC (Area Under the Curve) can be used to evaluate the trade-off between true positive rate and false positive rate, further informing the model’s performance.
Another important aspect to consider is the impact of undersampling on the model’s generalization capabilities. Cross-validation techniques, such as stratified k-fold or leave-one-out, can be employed to assess the model’s performance on unseen data and ensure the robustness of the findings.
Adapting Undersampling for Regression Tasks
While undersampling is primarily associated with classification tasks, it can also be applied to regression problems, particularly when dealing with imbalanced datasets. In regression tasks, undersampling can be used to address the issue of unequal representation of target values, which can lead to biased model predictions.
In the context of regression, undersampling can be used to balance the distribution of target values, ensuring that the model learns from a more representative sample of the data. This can be achieved by selectively removing instances from the majority target value range while preserving the overall distribution of the target variable.
By incorporating undersampling into the regression model evaluation process, practitioners can gain a more nuanced understanding of the model’s performance, considering not just the overall Mean Squared Error (MSE) or Root Mean Squared Error (RMSE), but also the Mean Absolute Error (MAE) and R-squared metrics, which provide additional insights into the model’s predictive capabilities.
Conclusion
Undersampling is a powerful technique in the machine learning arsenal, particularly when dealing with imbalanced datasets. By reducing the size of the majority class, undersampling helps to create a more balanced dataset, enabling the model to learn the patterns in the minority class more effectively. When combined with other techniques, such as oversampling or SMOTE, undersampling can lead to significant improvements in model performance, as measured by a range of evaluation metrics beyond just accuracy.
As with any model evaluation approach, it is crucial to consider the specific context of the problem and the characteristics of the dataset. Practitioners should carefully assess the impact of undersampling on the model’s generalization capabilities and adapt the
Machine Learning Model Evaluation: Beyond Accuracy
Comprehensive Model Assessment for Reliable Performance
In the realm of machine learning, the evaluation of model performance goes far beyond the simplistic metric of accuracy. While accuracy is undoubtedly an essential factor, a truly robust and reliable Machine Learning Model requires a comprehensive assessment that considers a multitude of evaluation metrics. This comprehensive approach ensures that the model’s performance is fair, unbiased, and can be trusted to make accurate predictions in real-world scenarios.
One critical aspect of model evaluation is the consideration of precision, recall, and F1-score. Precision measures the model’s ability to avoid false positives, while recall reflects its capacity to identify true positives. The F1-score, which is the harmonic mean of precision and recall, provides a balanced and holistic assessment of the model’s performance. By evaluating these metrics, practitioners can gain a deeper understanding of the model’s strengths, weaknesses, and overall effectiveness in handling different types of data and use cases.
Additionally, the analysis of Receiver Operating Characteristic (ROC) curves and the Area Under the Curve (AUC) can offer valuable insights. ROC curves illustrate the trade-off between the true positive rate and the false positive rate, allowing for a more nuanced understanding of the model’s performance across different decision thresholds. The AUC metric, which represents the probability that the model will rank a randomly chosen positive instance higher than a randomly chosen negative instance, provides a comprehensive assessment of the model’s discriminative power.
Furthermore, the use of confusion matrices can shed light on the model’s performance in terms of true positives, true negatives, false positives, and false negatives. This detailed breakdown of the model’s predictions can help identify areas for improvement and guide the development of more robust and reliable Machine Learning Models.
In the case of imbalanced datasets, where one class is significantly more prevalent than the other, techniques such as oversampling (e.g., SMOTE) and undersampling can be employed to ensure fair and reliable model assessment. These methods help to mitigate the inherent biases in the data, allowing the Machine Learning Model to be evaluated more accurately and fairly.
Cross-validation techniques, such as k-fold cross-validation and stratified k-fold cross-validation, further contribute to the robustness of model evaluation. These methods help to ensure that the model’s performance is assessed on a diverse and representative sample of the data, reducing the risk of overfitting and providing a more accurate estimate of the model’s true generalization capability.
For regression models, additional evaluation metrics like Mean Squared Error (MSE), Root Mean Squared Error (RMSE), Mean Absolute Error (MAE), and R-squared can be employed to assess the model’s performance in predicting continuous target variables. These metrics provide valuable insights into the model’s accuracy, precision, and the proportion of the target variable’s variance that is explained by the model.
By adopting a comprehensive approach to model evaluation, incorporating a diverse set of metrics, and addressing challenges posed by imbalanced datasets and other complexities, practitioners can develop Machine Learning Models that deliver reliable, fair, and trustworthy performance in real-world applications.
Evaluating Model Performance Beyond Accuracy
While accuracy is a crucial metric for assessing the performance of a Machine Learning Model, it is essential to consider a broader range of evaluation criteria to ensure the model’s reliability and fairness. By examining metrics such as precision, recall, F1-score, ROC curves, and AUC, practitioners can gain a more nuanced understanding of the model’s strengths, weaknesses, and overall effectiveness in handling different types of data and use cases.
In the context of imbalanced datasets, where one class is significantly more prevalent than the other, techniques like oversampling (e.g., SMOTE) and undersampling can be employed to mitigate the inherent biases in the data. This ensures that the Machine Learning Model is evaluated more accurately and fairly, providing a reliable assessment of its performance.
Cross-validation methods, such as k-fold cross-validation and stratified k-fold cross-validation, further contribute to the robustness of model evaluation by ensuring that the model’s performance is assessed on a diverse and representative sample of the data. This approach helps to reduce the risk of overfitting and provides a more accurate estimate of the model’s true generalization capability.
For regression models, additional evaluation metrics like MSE, RMSE, MAE, and R-squared can be used to assess the model’s accuracy in predicting continuous target variables. These metrics offer valuable insights into the model’s precision, the proportion of
Unlocking the Hidden Gems: Evaluating Machine Learning Models Beyond Accuracy
FAQ:
Q: What are the limitations of using accuracy as the sole evaluation metric for Machine Learning Models?
A: While accuracy is a commonly used metric for evaluating Machine Learning Models, it can be limiting as it fails to provide a comprehensive understanding of a model’s performance. Accuracy alone may not be sufficient, especially for complex problems or datasets with imbalanced classes, as it does not capture important aspects such as precision, recall, and the trade-offs between these metrics.
Q: How can Precision, Recall, and the F1-score be used to provide a more holistic evaluation of Machine Learning Models?
A: Precision, Recall, and the F1-score are valuable metrics that can provide a more in-depth understanding of a Machine Learning Model’s performance. Precision measures the model’s ability to correctly identify positive instances, while Recall measures its ability to identify all relevant positive instances. The F1-score is the harmonic mean of Precision and Recall, offering a balanced measure that considers both metrics.
Q: What are Receiver Operating Characteristic (ROC) curves and Area Under the Curve (AUC), and how can they be used to evaluate Machine Learning Models?
A: ROC curves and AUC are powerful tools for evaluating the performance of binary classification Machine Learning Models. ROC curves plot the True Positive Rate against the False Positive Rate, allowing you to assess the trade-off between sensitivity and specificity. The AUC, or Area Under the Curve, provides a single numeric value that summarizes the overall performance of the model, making it a useful metric for model comparison and selection.
Demystifying the Multiverse of Machine Learning Model Evaluation
FAQ:
Q: How can Confusion Matrices help in understanding the performance of Machine Learning Models?
A: Confusion Matrices provide a detailed breakdown of a Machine Learning Model’s performance by displaying the number of true positives, true negatives, false positives, and false negatives. This information can be used to gain deeper insights into the model’s strengths and weaknesses, and to identify areas for improvement.
Q: What techniques can be used to address imbalanced datasets when evaluating Machine Learning Models?
A: Imbalanced datasets can pose challenges in model evaluation. Techniques such as oversampling, undersampling, and SMOTE (Synthetic Minority Over-sampling Technique) can be used to address this issue. These methods aim to balance the class distribution, ensuring that the model’s performance is not skewed towards the majority class.
Q: How can Cross-Validation techniques be used to obtain reliable and unbiased estimates of Machine Learning Model performance?
A: Cross-Validation techniques, such as k-fold, stratified k-fold, and leave-one-out, are essential for evaluating Machine Learning Models. These methods help to ensure that the model’s performance is assessed on unseen data, providing a more accurate and unbiased estimate of its true capabilities.
Transcending the Accuracy Trap: A Comprehensive Approach to Model Assessment
FAQ:
Q: How can Regression Metrics like MSE, RMSE, MAE, and R-squared be used to evaluate the performance of Machine Learning Models for regression tasks?
A: For Machine Learning Models tackling regression problems, metrics like Mean Squared Error (MSE), Root Mean Squared Error (RMSE), Mean Absolute Error (MAE), and R-squared can provide valuable insights. These metrics capture different aspects of model performance, such as the magnitude of errors, the average deviation from the true values, and the proportion of variance explained by the model.
Q: Why is it important to establish baseline models when evaluating Machine Learning Models?
A: Establishing baseline models is crucial in the evaluation process, as it provides a reference point for assessing the performance of the Machine Learning Models being developed. Comparing the performance of the models against the baseline helps to determine whether the proposed models offer significant improvements or if they are merely matching the performance of simpler, more straightforward approaches.
Q: What techniques can be used for model comparison and selection, and how do they contribute to the overall evaluation of Machine Learning Models?
A: Techniques such as statistical significance testing, cross-validation, and holdout sets can be employed to compare the performance of multiple Machine Learning Models and select the most appropriate one for the given task. These methods help to ensure that the chosen model not only performs well on the training or validation data but also generalizes effectively to unseen, real-